Henry Fleischmann
h[fill in the gap]mann3 at gmail.com
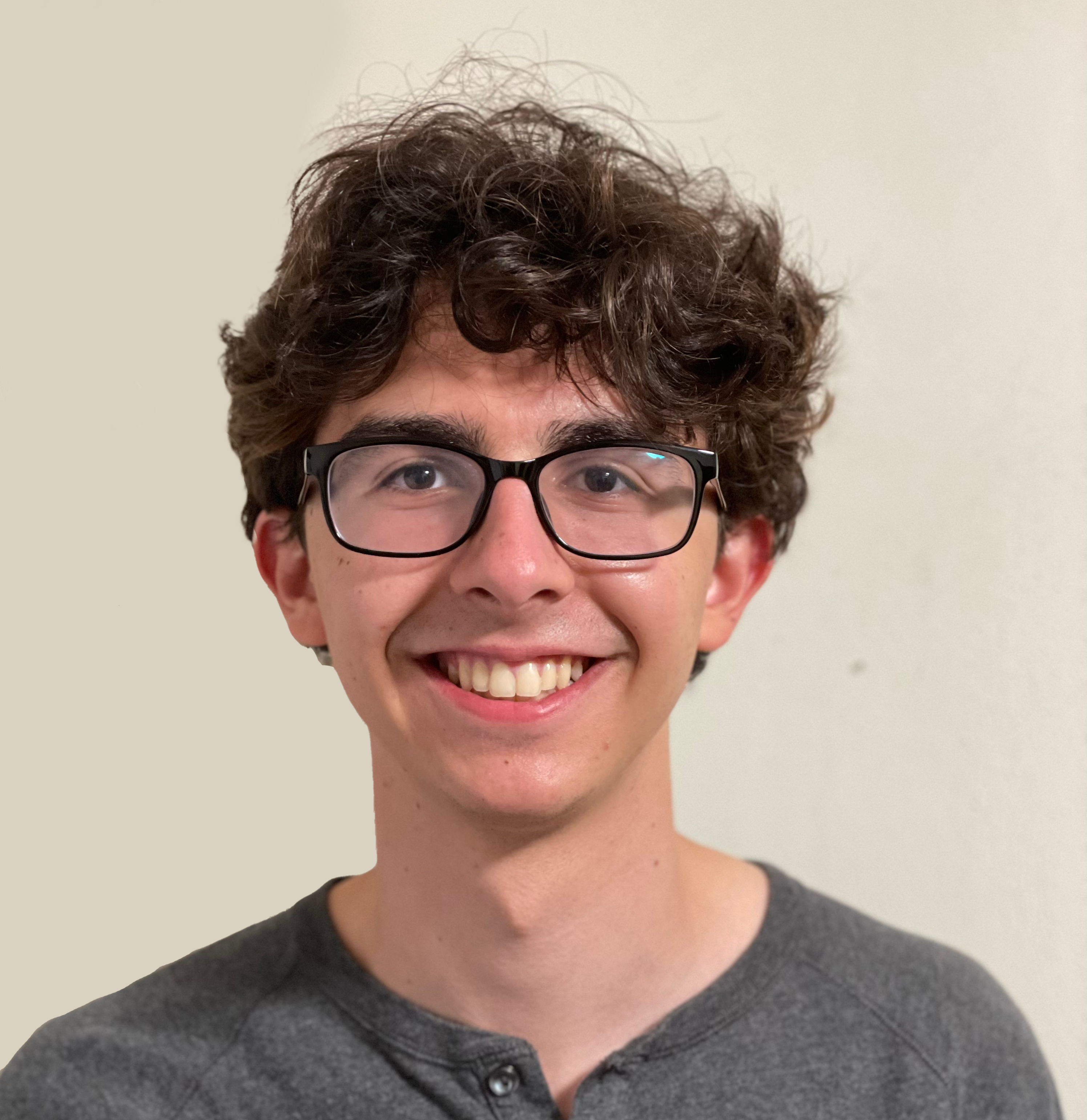
About Me
I graduated from the University of Michigan with highest honors in mathematics and highest honors in computer science. My honors thesis advisor was Greg Bodwin. While I am broadly interested in theoretical computer science and combinatorics, I am particularly passionate about extremal problems in discrete geometry, graph theory, and applying theory to make a social impact. During the 2023–2024 academic year I am at the University of Cambridge, studying for the MASt in Pure Mathematics as a Churchill Scholar. I will begin my Ph.D. in Computer Science at Carnegie Mellon University during Fall of 2024.
During the summer of 2023, I was a graduate mentor at the 2023 DIMACS REU program, working with Karthik C.S. and his students (Ashwin Padaki, Styopa Zharkov, and Kyrylo Karlov) on hardness of approximation of clustering objectives. I also mentored the students in his other project (Guillermo Gamboa, Josef Matějka, and Jakub Petr), constructing optimal Euclidean Steiner trees for the regular simplex.
I did my honors thesis with Greg Bodwin at the University of Michigan, devising sublinear time algorithms for constructing adjacency oracles of sparse spanning subgraphs, $k$-connectivity oracles, and multiplicative distance spanners. We also showed several lower bounds for our model.
During the 2021 and 2022 academic years, I worked on quantifying the fairness of the redistricting plans drawn in the new redistricting cycle. I began as a student researcher in LoG(M) , advised by Tim Ryan and Samuel Hansen. With LoG(M), I developed a Python script for quantifying redistricting fairness in Michigan and provided feedback to the Michigan Independent Citizens Redistricting Commission on their initial practice maps. Later I became a policy analyst for CLOSUP at the Gerald R. Ford School of Public Policy. As a policy analyst, I worked with Gregory Herschlag and Jonathan Mattingly, processing Michigan geographic shapefiles and election data to apply a modified version of the Multi-scale Merge-split Markov chain redistricting fairness algorithm. Alongside Jon X. Eguia, I also quantified the fairness of every redistricting plan in the 2022 cycle according to simpler measures, co-authoring the IPPSR Partisan Advantage Tracker and issuing an associated policy report.
In the summer of 2021, I participated in the SMALL REU program at Williams College. At SMALL I was part of Steven J. Miller's Number Theory and Probability Theory group, and I worked on four subprojects spanning discrete geometry, random matrix theory, additive combinatorics, and combinatorial game theory. The discrete geometry subproject was co-advised by Eyvindur A. Palsson and Charles Wolf.
During the summer of 2020, I participated (virtually) in the Extremal Graph Theory and Dynamical Systems REU at Rochester Institute of Technology. I studied the existence of efficient $(j,k)$-dominating functions on graphs with Brendan Rooney.